Eyeson AI Adapter
Overview of the Eyeson AI Adapter
The Eyeson AI Adapter architecture consists of two essential components:
- The Eyeson API endpoint group
forward stream
, which manages the streaming data transfer - A customer-implemented AI Module that processes the streams and returns layout instructions or enhanced video/audio content
These components work together to enable real-time AI processing of video conference streams while maintaining seamless integration with the Eyeson platform.
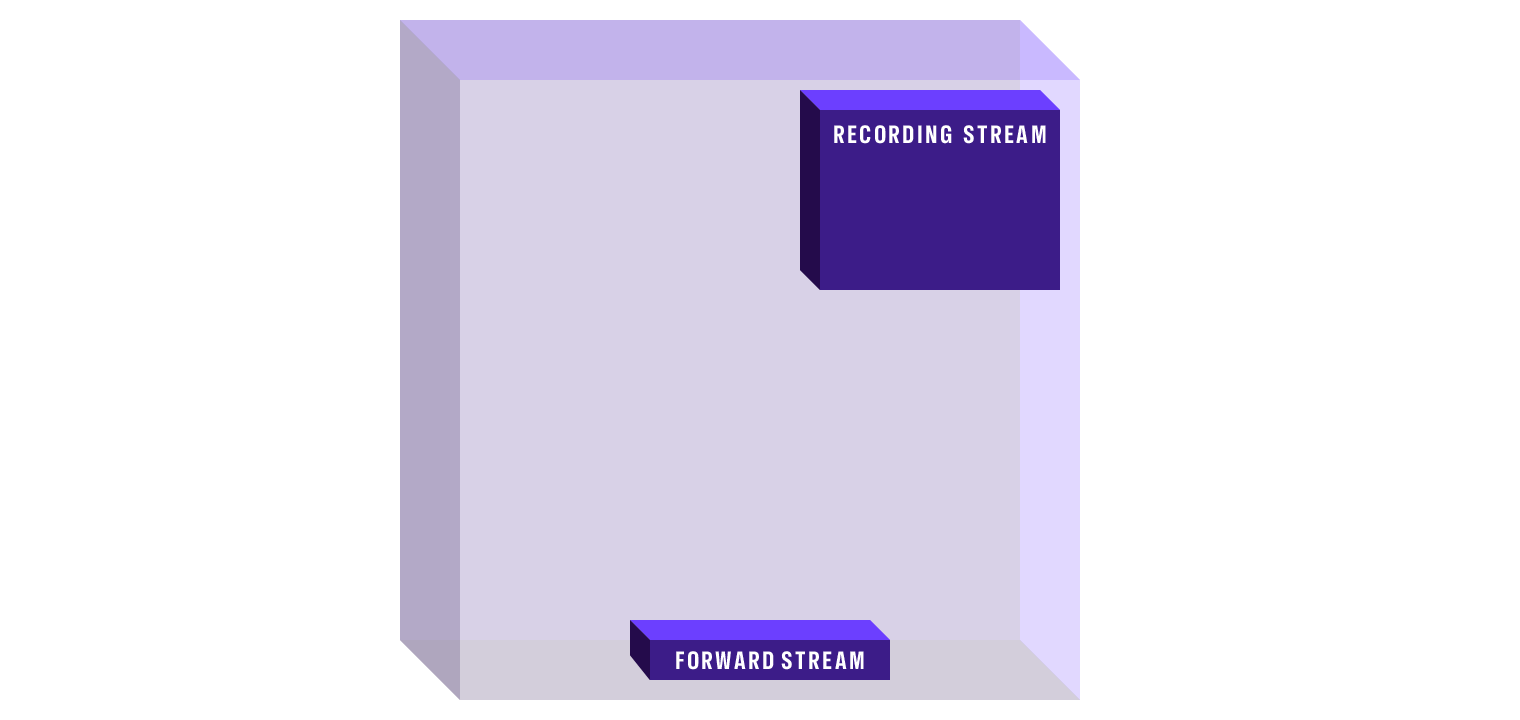
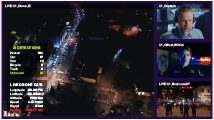
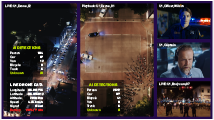
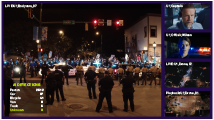
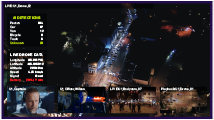
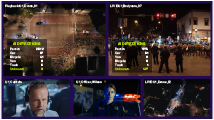
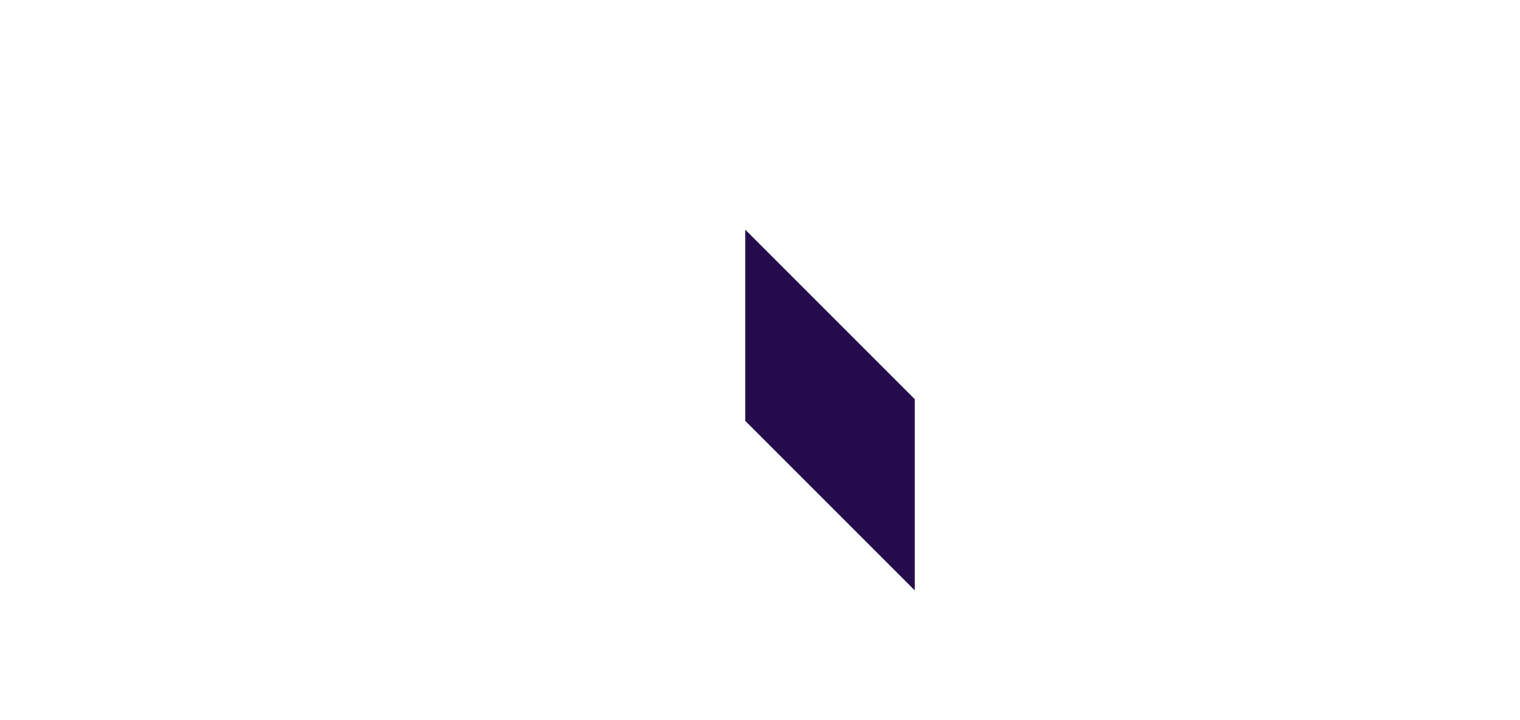
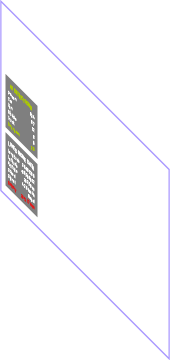
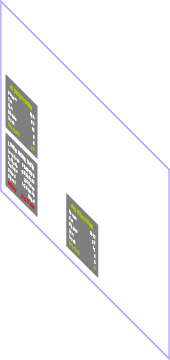
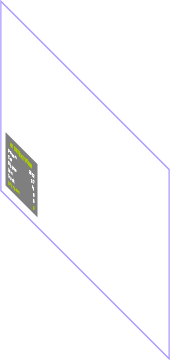
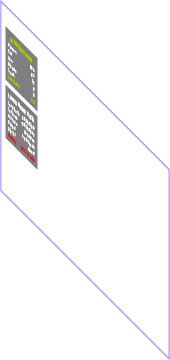
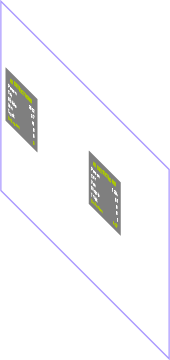
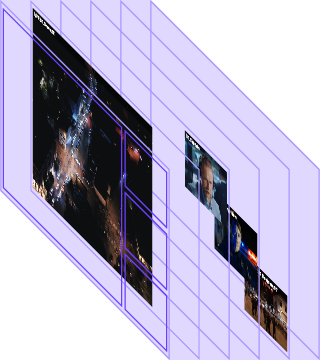
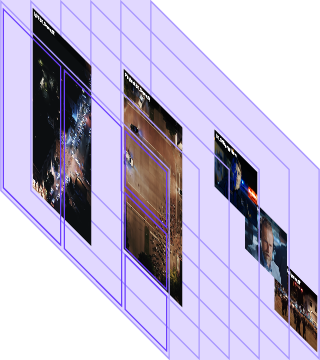
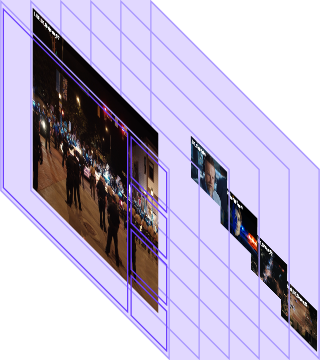
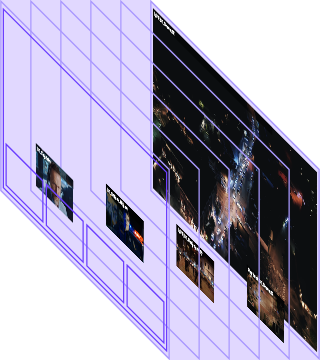
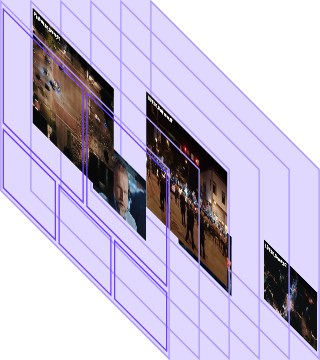
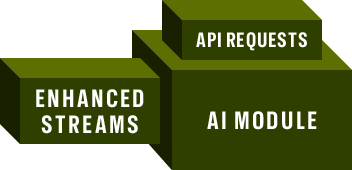
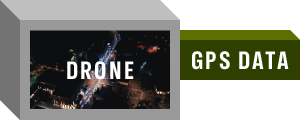
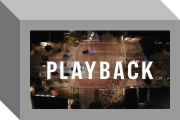
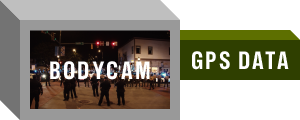
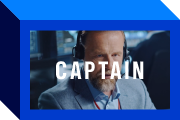
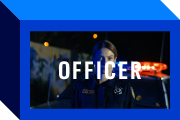
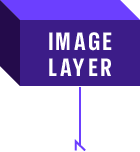
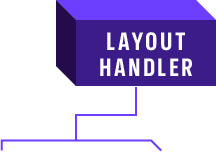
The diagram above illustrates the stream forwarding process. When a POST
request is sent to the /forward/source
endpoint, it initiates the forwarding of video/audio streams to the URL of the WHIP
server specified in the request parameters. The streams at the top of the diagram are unidirectional (send-only), while the streams at the bottom support bidirectional communication (send/receive), enabling both data transmission and reception.
At the ingress of your AI module, you must process the incoming stream data (image and/or audio) to prepare it for analysis within your AI pipeline. This typically involves decoding the stream, extracting the relevant frames or audio segments, and formatting the data according to the requirements of your AI processing algorithms.
Streams are forwarded in their native format without any modification or transcoding, preserving the original quality and characteristics of the audio and video data. This ensures maximum compatibility with your AI processing pipeline while maintaining the integrity of the source material.
At the end of the AI pipeline, you have the option to implement various actions that can be transmitted to the API as commands. These actions enable real-time response to the AI analysis results and can be integrated with other Eyeson functionalities.
- Triggering an alert and setting an overlay.
- Changing the layout and moving the focus to a certain stream.
- Writing a transcript to a file
- Adding a screen capture to a report
- a.s.o.
The system architecture provides flexible stream routing capabilities, allowing you to:
- Direct multiple source streams to a single AI pipeline for consolidated processing
- Send a single source stream to multiple AI pipelines for parallel analysis
- Create custom combinations of stream-to-pipeline mappings
This flexible routing approach enables you to optimize your AI processing based on available bandwidth and specific analytical requirements, ensuring that each source stream receives the most appropriate intelligence processing.
When a source stream stops delivering data, the corresponding forward command will automatically terminate. To maintain continuous stream forwarding, you must implement monitoring in your ingress system to detect disconnections and automatically reinitiate the forward command when needed.
AI Integration Example
Modern video communication systems are increasingly incorporating AI capabilities to enhance functionality and user experience. This section examines different approaches to AI integration, from device-level implementation to sophisticated multi-AI architectures.
Stage 1: Compressed Video
Here are the compressed sources evaluated and selected for the next stage, where the Video Processing Unit detects changes in the content and flags them for further processing, as shown below.
Stage 2: Uncompressed Video
The Object Detection Model (ODM) analyzes and annotates the uncompressed versions of the selected sources.
{
"ipcam001": {
"time":"2025-04-10T13:07:35Z",
"detections": {
"detection-id-1": {
"class": "person",
"score": 0.87,
"box": ["x1","y1","x2","y2"],
},
"detection-id-2": {
"class": "bag",
"score": 0.64,
"box": ["x1","y1","x2","y2"],
},
}
...
},
"ipcam002": {
"time":"2025-04-10T13:07:35Z",
"detections": {
"detection-id-3": {
"class": "person",
"score": 0.87,
"box": ["x1","y1","x2","y2"],
}
}
...
}
}
Stage 3: Additional Intelligence
For improved scene understanding, results of the ODM, along with other sensor data and intelligence, are analyzed using a Large Language Model (LLM). The LLM's output may include a scene description, a step-by-step plan, or high-risk statistics.
{
"security": {
"time": "2025-04-10T13:07:35Z",
"door_access_system_status": "operational",
"fire_alarm_system_status": "active",
"smoke_detector_status": "N/A",
"intrusion_alerts": 0,
"security_camera_status": "all cameras active",
"security_cameras":{
"ipcam001": {
"status": "active",
"time":"2025-04-10T13:07:35Z",
"frame rate in fps": 25,
"resolution": "1920x1080",
"bitrate in kbps": 2048,
"network latency in ms": 243,
"packet loss in %": 0.2
},
...
}
},
"occupancy": {
"total_occupants": 120,
"current_floor_occupancy": {
"floor_1": 56,
"floor_2": 21,
"floor_3": 43
}
},
...
}
Stage 4: Composed Video
Based on the ODM results all video sources are ranked in priority and a layout is generated for Eyesons layout endpoint. The various streams are then forwarded to Eyeson which merges them into a single video stream.
$ curl -X POST \
-d "name=custom-map" \
-d "map=[ \
[0, 0, 1280, 540, "cover"], \
[0, 540, 426, 180, "cover"], \
[426, 540, 426, 180, "cover"], \
[852, 540, 428, 180, "cover"] \
]" \
-d "users[]=ipcam001" \ #ipcam with most detections
-d "users[]=ipcam004" \
-d "users[]=ipcam003" \
-d "users[]=ipcam002" \
-d "layout=custom" \
"https://api.eyeson.team/rooms/$ACCESS_KEY/layout"
Based on the LLM results a overlay is generated and send to Eyesons layers endpoint. For a detailed example read How to Update Overlays. The generated layout and overlay provides a seamless view of the scene by integrating feeds such as Drone video, body-mounted cameras, and collaboration cameras.
Stage 5: Distributed Interactive Video
AI, video sources (such as drones, bodycams, and sensors) can operate autonomously, but with human operators in the loop (HITL), the process becomes much more effective.
Advanced Implementation: Multiple AI
The most sophisticated implementation involves an Edge MCU architecture that integrates multiple AI systems. This model provides several key advantages:
- Distributed AI Processing
- Real-time Stream Processing
- Intelligent Content Routing
- Enhanced Collaboration Features
The architecture follows this structure:
This configuration allows for:
- Parallel AI processing
- Intelligent stream filtering
- Dynamic layout switching
- Real-time content enrichment
- Immediate collaboration integration
The multi-AI approach provides greater flexibility and functionality compared to single-AI implementations, while maintaining the efficiency benefits of edge computing.
AI Model suggestions for Edge AI
Here's a table giving you ideas on your options for Edge AI:
Datasets | Examples of Models | Use Cases and benefits |
---|---|---|
Compressed Video | ● MobileNet Lightweight motion detection ● Coviar action Recognition | Real Time Actions or motion detection ● Streams filtering and prioritization -> Layout API ● Smaller dataset for lower CPU and Energy consumption |
Decompressed Video | ● YOLO - Object Detection ● C3D - Motion and object detection ● I3D - Action Recognition ● ViT - Image classification ● CLIP - Image captioning | Real Time Complex actions detection ● Streams filtering and prioritization -> Layout API Real Time Complex objects detection ● Streams filtering and prioritization -> Layout API ● Content enrichment (visual tagging, image captioning) ● STAGED AI: Streams Routing to cloud LLM for additional analysis |
Composed Video | ● HMFNet ● V-JEPA - Layouted Video + Audio + Metadata. One composed uncompressed real time stream of multiple synchronised sources | Real Time Scene understanding ● Predictions and recommendations -> Event predictions, live recommendations, automated additional content collection or suggestions (updated maps, additional sources) from LLMs ● Streams Routing for escalation to LLMS Real Time Data quality augmentation ● X synchronised sources: X videos with different angles in 1 video stream (targeting) |
Individual or mixed Audio | ● NLP Models: ● STT ● TTS ● Sentiment Analysis | Real Time Content Enrichment ● Voice Transcription, Translation, Sentiment analysis, sounds analysis Layout Piloting and streams routing ● Voice activation, Key words |
Relevant links
- /rooms/
ROOM_ID
/forward/source
This endpoint allows the forwarding of one source stream to an AI ingress. - /rooms/
ROOM_ID
/forward/mcu
This endpoint forwards the whole One View, you would use this for transcription or reasoning. - /rooms/
ROOM_ID
/forward/playback
This endpoint allows the forwarding of a playback.